Shall we start from Bach? Or why and how in silico modeling can help to assess xenobiotics exposure in CNS
Physiologically based pharmacokinetic (PBPK) modeling serves as a powerful tool for predicting drug distribution in the central nervous system (CNS). By integrating experimental and computational data, Professor Sebastian Polak explores how xenobiotics interact with the blood-brain barrier (BBB) and blood-CSF barrier (BCSFB). His work leverages mechanistic modeling, in vitro-in vivo extrapolation (IVIVE), and quantitative structure-activity relationships (QSAR) to simulate drug transport, metabolism, and neurotoxicity. This approach enhances our understanding of CNS pharmacokinetics, supporting drug development and regulatory decision-making.
This video is licensed under a Creative Commons Attribution-NonCommercial-NoDerivatives (CC BY-NC-ND) license. You are free to share it for non-commercial purposes with proper attribution, but no modifications or adaptations are allowed.
1. Why use this method?
The in silico modeling method aims to predict the pharmacokinetics of xenobiotics in the central nervous system (CNS) by integrating physiologically based pharmacokinetic (PBPK) models with experimental and computational data. This approach accounts for passive and active transport mechanisms, enzymatic metabolism, and interindividual variability in transporter expression at the blood-brain barrier (BBB) and blood-CSF barrier (BCSFB). By combining quantitative structure-activity relationships (QSAR), in vitro-in vivo extrapolation (IVIVE), and mechanistic modeling, this method enables accurate simulation of xenobiotic distribution, metabolism, and potential neurotoxicity, supporting drug development and regulatory decision-making.
2. What you’ll need
- Physiologically Based Pharmacokinetic (PBPK) model tailored for the CNS
- In vitro ADME/PK (metabolism, permeation, affinity to transporters – e.g., P-gp, BCRP, OATP1A2, OATP2B1), Phys-chem, formulation data for the compound of interest
- In silico modeling software
- Data on transporter abundance and activity in brain microvessels
- Human and preclinical species’ pharmacokinetic and brain exposure data – for model validation
3. Step-by-step instructions
A. Preparation of the Pharmacokinetic Model
- Integration of physicochemical and ADME/Tox data for the studied xenobiotic
- Incorporation of transporter parameters across the BBB and BCSFB
- Scaling of in vitro transporter data to in vivo conditions using intersystem extrapolation factors
- Calibration of the model based on available experimental data and transporter abundance measurements
- Validation of the model by comparing it with literature and experimental data
B. Simulation and Data Analysis
- Conducting simulations of xenobiotic distribution and permeability in the CNS
- Evaluating compound concentrations in key brain regions (plasma, cerebrospinal fluid, intracellular and interstitial fluid)
- Assessing the impact of active transport mechanisms (influx and efflux transporters)
- Analyzing metabolism and potential neurotoxicity
- Performing sensitivity analyses for key parameters (e.g., transporter activity, passive diffusion, metabolic clearance)
- Exploring interindividual variability in transporter expression and its impact on drug exposure
4. Practical tips
- Models should distinguish between different brain compartments (plasma, CSF-cerebrospinal fluid, ISF-interstitial fluid, ICF-intracellular fluid) to capture xenobiotic distribution accurately
- Accounting for active and passive transport mechanisms is critical for accurate predictions
- Calibration of model parameters is crucial for result reliability
- Interindividual variability in transporter abundance should be considered in simulations
- Validation against available human or preclinical species data enhances model credibility
5. Critical appraisal & implications for future research
In silico modeling is a powerful tool for predicting xenobiotic pharmacokinetics in the CNS. Potential limitations include limited access to certain experimental data, the need for precise transporter activity measurements, and possible errors due to mathematical model simplifications. Further methodological development improved experimental validation and can significantly enhance prediction accuracy. Collaboration between experimentalists and modelers is crucial to improving model accuracy and applicability.
This protocol is licensed under a Creative Commons Attribution-NonCommercial (CC BY-NC) license, allowing sharing and adaptation for non-commercial purposes with proper attribution.
Send a message
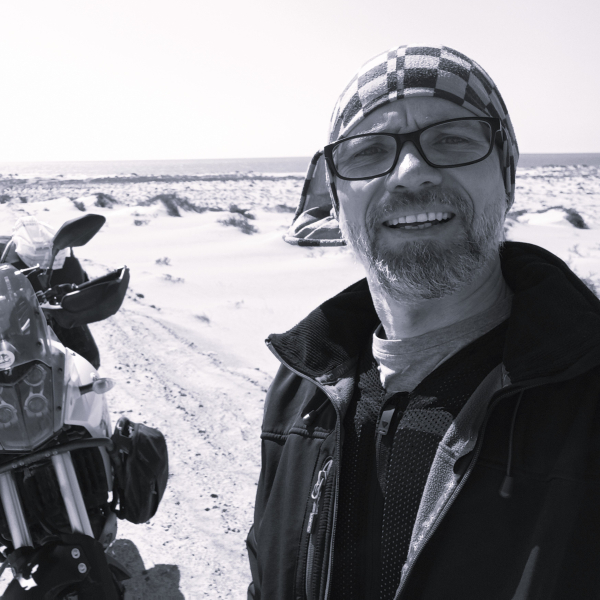
Adding comments is only possible for registered users.
Sign in